31
Mar 24
03:54
Brainstorming on why it’s still impossible to use deep learning to learn the Fast Fourier Transform
The Fourier transform allows one to take a time domain signal and turn it into frequencies with no loss of information. It’s still at the front end of the vast majority of speech and audio ML systems, despite some pressure from learned kernels like TasNet. The complexity of the fast fourier transform is N log N, and interestingly, we can’t beat this yet with deep learning approaches. This post is a collection of shower thoughts on this.
It’s a fair objection to say that a shallow linear network form of an FFT is not deep enough to be deep learning, but we don’t have many neural frontends that clearly outcompete the FFT either, and they are typically more computationally complex. The exception might be TasNet, which learns overcomplete basis functions of short length. But this a different application where symmetry and perfect reconstruction is not needed. The spirit of this post is just about how a deep learning approach would take advantage of something *like* the structure of an FFT, which seems to be optimal and difficult to get to.
The question is still open whether there is a way to learn computationally optimal solutions with deep learning, but it is clear that it is not something we know how to do yet for the general case because of the way models are designed and trained. Rectangular neural network layers are problematic for this purpose because they are a static shape. They are convenient for GPUs and TPUs because at the core they are good at rectangular matrix multiplication. We have ‘fake’ sparsity on these by using zeros in the matrix, but we still multiply them. This is very different from even 15 years ago, when sparse matrix representations on CPU needed to skip the zeros out of necessity. Good and clever tradeoffs like block sparsity and low rank multiplication exist, but again, these are all about keeping things rectangular.
This is interesting to me on some deep level – it makes me recall a bit of the debate in that happened in early AI at Dartmouth lore in the 50’s/60’s. Minsky vs Rosenblatt involved one person showing how powerful stacked linear layers were, and was devastated when the other tore him down with an XOR function and stole his girlfriend at the same time. The similarity is not the drama (which I hope someone could give me more info on, because I’ve forgotten a lot of it), but the obvious and vulgar XOR function being problematic for the linear NN is sort of like how we can do very complicated things with DNNs now but we can’t learn an FFT for the life of us. And just as there was a non-linear activation function that solved the XOR problem, I wonder what it would take to get us to be able to learn an FFT.
FFTs are a mostly solved problem, and deep learning system that learns one won’t be changing the world because of its FFT implementation. But a system that could learn an FFT probably would be useful for a lot of other problems where we don’t have a solution yet.
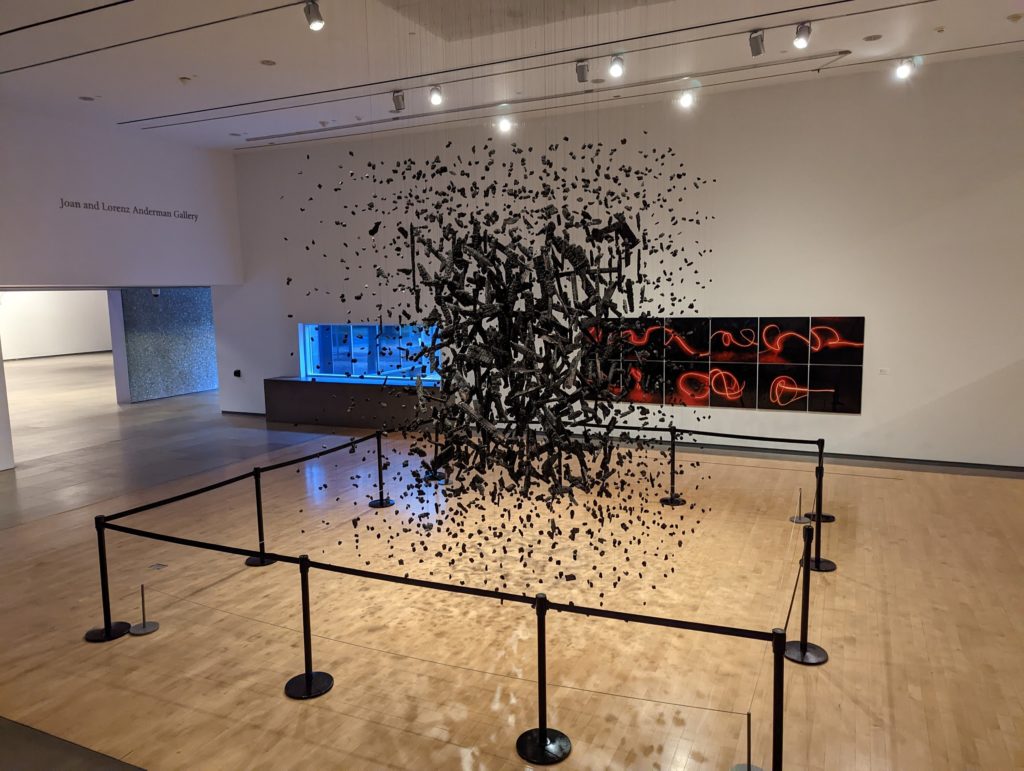
Have you ever seen one of those 3-D art pieces that are constructed of hanging bits that appear formless unless you look at it from a certain angle, where some interesting image appears? This is one from the modern art museum in Phoenix (unfortunately I forgot who the artist or piece title was and if even had an optimal angle, but this gives an idea). This is not far off from what we’re asking of the DL process, if you let the image be even more scrambled in 3d. Just as moving around the 3D object gradually changes your view’s projection matrix. But for the most part there is really no smooth gradient unless you are within a few inches of the optimal viewing angle. The question is, are there some transformations you could do from far-from-optimal positions that would give a gradient? My completely ignorant intuition says there might be some useful transformation you could do with a subspace or subset of the image that would unlock a gradient, but this is something that requires more thought and is probably a problem people have worked on before. If you know of something like that please drop me a line.
There is a clear trend in the research that data and scale is mostly the priority, and we should focus our efforts on larger, more interesting things. But we should invest some time into learning the limits of the learning, if you will. The rest of this post is going to be increasingly rambling, because these are unstructured notes on the topic.
Consider the discrete Fourier transform. It’s easy to implement the DFT in N**2, but N log N exists as the FFT. It’s objectively better in all regards except for readability and difficulty writing it.
As a reminder for why the FFT is faster:
- Take the sinusoid at frequency f multiplied by the input signal, and let’s call that sin_f() = sin(t * f * 2pi) * x(t).
- sin_2f(t) has the same sin_f(t) at t==0, so you don’t need to recompute that.
- Note that this is obvious because sin(0*f*n)*x(t) is zero since sin(0) is 0 – the key is realizing where the harmonic sinusoids overlap with the sinusoid at fundamental frequency.
- For any integer n, 2nf shares the same value at t=0, t=.5, (assuming t=1 is the full time window).
- For 4nf, it’s t=0, t=.25, t=.5, t=.75.
- There aren’t going to be symmetries for 3nf other than t=0 between 3nf and f, but these exist between 3f and 3nf.
- This is equates to a lot of values we don’t need to recompute. The highest frequencies have almost all of their values filled in.
Also caching the sinusoids into a lookup table, or their ‘twiddles’ would be valuable here. The cos() component can also use the same table. Note that we have to be selective with what symmetries we exploit here – for many frequencies only the first quarter of the sinusoid is actually required because of the symmetry that repeats every pi/2, but there’s no savings there since that’s just one-time compute that will need extra computation to restore.
This process is done by manual inspection. Could chat gpt realize this? It’s possible. Could a modern neural network take advantage of this? It seems very difficult. There’s no incentive for the network to do this, and the gradient seems very uncertain between the traditional n**2 DFT matrix and the FFT one that is sparse.
So one question is how to incentivize the network to use sparse and reducible structures. L1/L2 regularization is a hack that won’t get us there because of this gradient issue.
I remember coming across the following paper in 2018 which looked at just this, but did not propose solutions.
ARE EFFICIENT DEEP REPRESENTATIONS LEARNABLE? – 2018 ICLR MIT – tries to learn sparse FFT with L1 reg. And of course fails for the reasons I mentioned, even when initializing with near FFT weights after a noise threshold, it’s not able to recover.
Note the steep cliff at the loss that shows this gradient issue.
The idea of reference is not typically built into NNs, but maybe should be. The FFT’s case is interesting because the structure of reference exists, but is complicated, related to harmonics, giving the famous butterfly structure.
Efficient Architecture Search for Diverse Tasks (CMU) 2022 neurips – uses fft, doesn’t learn it.
Do deep neural networks have an inbuilt Occam’s razor? – 2023 arxiv, oxford. Doesn’t do FFT, but asks for bayesian posterior for these networks appearing in efficient forms. Claims success of modern DNNs how implicit occam’s razor. Has method of artificially reducing compute.
Achieving Occam’s Razor: Deep Learning for Optimal Model Reduction – SUNYSB 2023 – uses bottleneck to achieve minimal parameters. I think the idea is to call the bottleneck a minimal representation that can discard the previous layers as a representation. This is interesting, but not exactly novel. How do they show the minimal parameters? It doesn’t appear have an independence term. But more to the point, this doesn’t deal with the complexity involved in the transform to or from the representation, which is kind of my focus here.
It’s Hard For Neural Networks to Learn the Game of Life 2021 International Joint Conference on Neural Networks (IJCNN) Los Almos. This is fun because there is the time component in game of life. Looks at it from the lucky random initial weights (lottery tickets). Finds rarely converge. Needs much more parameters to consistently converge. Minimal architectures fail with slight noise. It’s hard to implement the joint sampling, so I’d recommend they do a single cell prediction only and see how that performs (the network can still take the 32×32 board as input, just predict the middle cell).
The boolean problem is interesting because it has to be sharp – predictions should be logits and sampled, probably. But then, it should be jointly sampled, or if sequential, conditionally sampled to be sharp. I don’t think they did that here, so it’s not surprising the result is bad (i.e. each cell is independently evaluated based on a logit value that is unconditional)
The other major issue is colinearity. It’s easy to learn the same predictor twice and split it into two weights, which have infinite solutions (x + y) = k. The only mechanism discouraging this is that doing this is not efficient – it restricts the amount of information that the network holds, since it’s possible to reduce x and y to a single weight x + y. But here’s the problem, if x and y are not near zero, it’s very hard to reduce this, and the gradient won’t help. L1 regularization will be indifferent to this, and L2 will actually encourage this kind of wastefulness, because it is the lowest when x = y = .5 k due to disliking larger values like x = k, y = 0. (to be clear, L2 with x = y = .5k is 2(.5k)**2 = .5k**2 vs L2 with x=k;y=0 is k**2). If you squint, this is similar to the sparsity/efficiency learning problem.
These challenges may appear somewhat against the direction of the lottery ticket hypothesis, but it doesn’t contradict it. The lottery ticket hypothesis says that there is an advantage to train subnetworks with certain initializations. It says nothing about finding the optimal solution. In fact, it shows evidence that training NNs the ‘standard way’ without lottery tickets produces results that are quite far from a better but non-optimal solution.
Genetic algorithms do seem promising for their ability to subdivide problems, and the ability to have variable length chromosomes.
Genetic programming was popular before and was a code solution. Let’s see where they’re at.
Explainable Artificial Intelligence by Genetic Programming: A Survey 2022 IEEE transaction on evolutionary computation – examples are typical math operator/function but also has image classification!. Has a nice table of real world applications.
Deep learning typically only takes sequences via autoregression or attention. These struggle to refer past a certain window. Hierarchy and reference seems like one solution here. Reference is built into attention, but only applies to the input. In theory we’d like layer-free reference
There is also the concept of optional residual layers to scale complexity. The layers can reuse the same weights, or have new ones.
So let’s take code generating LLMs. Assume the FFT has not yet been invented and you trained only in this world. It should be possible to see the sparsity of the problem by using caching in the data to infer the structure of the new algorithm.
Now let’s consider only a subset of LLM that uses tokens to represent assembly language code, or even object code. Operations, constants, memory addresses for functions. We could make things easier and add references by allowing functions, and possibly providing a library of common functions like print, read, memcpy.
In theory this makes the training easier than a high level program language with the reduced vocabulary. But in practice, who knows. We need a compiler that deals with this to handle the function tokens, since that’s not exactly visible in the bytecode except for push/pop/jump. My guess is the part about LLMs being pretty bad at math will only compound in this kind of problem. The other code generation research and projects are probably worth looking into, because they are aware of this. AFAIK, there’s still nothing that can do high level consistency, but simple and contained problems like leetcode are ~70% there. These are often very small, single function programs that have some computational complexity in them. Because it’s small, there isn’t as much chance for the consistency to fail as in a larger program. But even then, the techniques involve post-training fixups like just generating many solutions, testing them, and discarding the incorrect ones.
What is a fun simple model I could write now? Let’s assume the problem is ‘echo’ that appends ‘!’ to the end – it’s non trivial to generate the assembly needed to carry the input to the output. The easiest program to do is just hello world of course. How would you train for such a program? With the traditional LLM paradigm, the training input is for all possible programs, provide a lot of possible inputs and assembly that can generate the desired output (note that the program output isn’t required, but might help). Then you somehow at inference pass the model the input/output pairs for your hello world program, and ask it to generate the assembly or object code.
But of course, this generalization comes at a cost that is probably too high for today’s compute, so perhaps it’s better to focus on a subset of programs. And even then, it’s not clear we’d get the optimal program even if it was correct. To get an efficient program, we either have to rely on regularization, penalizing complexity, or hope that the inductive bias from efficient programs will create efficient programs. The other way to do it is to train on various versions of the program that go from less efficient to more efficient, and have the model be aware of this.
Another consideration is how premature it is to worry about optimizing towards the ‘clever’ solutions like FFTs, and problems that have some beautiful symmetry that can be exploited for efficiency. How many actual problems have these clean behaviors? My intuition here is that most problems are not as ‘beautiful’ as the Fourier transform, but that it’s still useful to consider now, because basic things like colinearity and excess architecture size that we know exist in DNNs are strongly connected to this concept – we don’t just want to exploit symmetry, we want to reduce unnecessary asymmetry. The FFT is a nice target that encapsulates both these problems because we have infinite data, is scalable, and has a well understood symmetry.
So let’s get started with the echo example, and assume we have a syscall for print and leaving out string operators for now. There are many ways to write this, but the key is to iterate over the input, copying and printing a character each step. The loop should be a check for the null terminator, followed by an increment pointer, load char from memory to the register, print the register, and then jump back to the start.
Often, we want program correctness first, and efficiency next. But this is not always the case. Consider audio codecs, which are usually lossy because we want a nice balance of efficiency and correctness, even if the correctness is above the threshold. Since it’s hard to get DNNs to be exact for continuous output, (even learning the identity function with zero error is basically impossible), the balance of correctness and efficiency seems better suited for DNNs without special tricks.
If you think about this long enough in the context of genetic programming, it’s possible to see the (flawed) logic in intelligent design advocates against evolution. Irreducible complexity in biological systems is a mirage that disappears when you look closely. And in fact we have rods and cones in front of the optic nerve that reduce visual quality for no good reason other than something analogous to the random initialization that put them there, and it was hard to rearrange them. However, I think it’s a fair argument to suggest that certain complexities aren’t approachable by certain systems. Evolution is sufficient to create humans, but the FFT might be out of reach for the current deep learning paradigm.
Conceptually, the idea of replacing a value that is computed the same way is the idea of referencing another value (that is sin_2f(0) should use the value computed by sin_f(0)).
Hyperparameter search like vizier or even grid search are one way of trying to identify the best tradeoff rate-distortion curve. Network architecture search has seemingly lost steam from when it was in full force a few years ago 2018-2021 seemed like a peak. But even these methods were fairly rigid and probably wouldn’t allow the architectural changes to get to the FFT.
All this is to say, I still don’t know of a concrete method to approach this problem, but the concept of learnable references is something that seems like one area that deep learning has trouble with. We can hardcode the architecture to reference other values, such as we do with residual networks or skip connections, but we don’t have a great way of doing this across the entire network, or even within the same matrix. This is not my area, so there’s also a good chance I’m missing some important research here. But it does also seem like a fun area to work in.